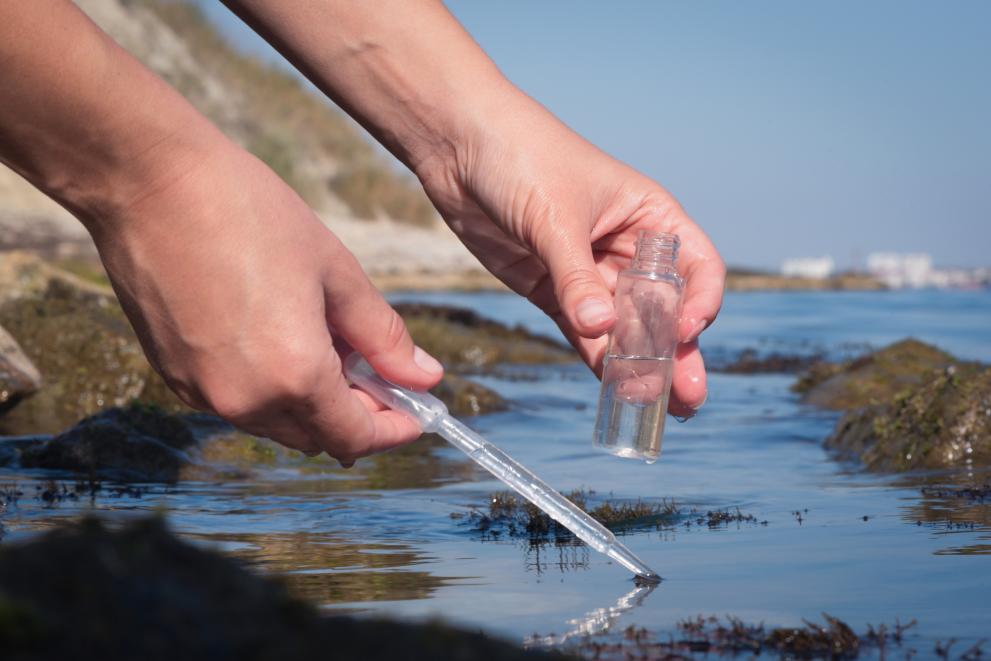
There is an increasingly large amount of data available – for example from weather monitoring – that may be able to inform environmental risk management. Harnessing this data, however, requires sophisticated methods that can link complex sets of variables. In other words, it is difficult to predict the frequency and strength of natural hazards, since they are functions of the interaction of multiple phenomena.
Data-driven forecasting models – which use statistical relationships and algorithms to make predictions – must also rely on data which may be incomplete, inconsistent and sparse in the case of rare events. Nevertheless, they can be effective, and can be improved by drawing on the increasing volumes of data from sources such as remote sensing, monitoring stations and sampling. Data-driven hazard forecasting has now been developed for flooding, air pollution and harmful algal blooms, for example.
Researchers in the US have applied this method to recreational beach water quality. Due to the potential health hazard posed by faecal contamination of beach water, water quality is monitored in many parts of the world for faecal indicator bacteria (FIB). If detected, enteric pathogens such as Escherichia coli or norovirus may be present. Monitoring is generally infrequent, however – it is conducted at most weekly, and laboratory analysis takes up to 48 hours. This means there is a delay between bacteria being detected, beach management decisions, and public notification – and interventions may not even reflect current conditions.
In California, where this study was carried out, models currently used to predict when FIB levels will exceed safety standards tend to only offer information on the persistence of bacteria following detection – or real-time information (“nowcasts”) – rather than indicating future conditions. Nowcast models are used at many marine and freshwater sites around the globe (e.g. US Great Lakes, Hong Kong, New Zealand and UK). They provide more frequent and more accurate information about beach water quality than sampling programmes alone can provide. Such short-term, same-day predictions give managers little time to make beach management decisions. It would be more useful to have forecasts of water quality looking ahead several days, but so far studies have only looked to predict bacteria levels up to a day ahead.
Using historical observations of FIB and environmental data from two California sites, Cowell Beach and Huntington State Beach – both popular with beachgoers – the researchers therefore looked to develop models that could effectively forecast when bacteria levels might exceed safety thresholds. (Although the study is US-based, the method could be applied to European sites1.)
The study drew on weekly and bi-weekly sample data on bacteria E. coli and Enterococcus from April-October in 2007–2021. Environment data included information on waves and water temperature from monitoring buoys, and accurate tidal predictions from the National Oceanic and Atmospheric Administration (NOAA). Nearly 270 environmental variables were involved in the model development, refined to fewer, most relevant variables as model training progressed – for example tide level was found to be especially important.
The researchers used four types of machine-learning model (e.g. ‘random forest’, ‘gradient boosted machine’), which worked to refine the importance of each variable for predicting whether the beaches would exceed regulatory standards for FIB. A total of 384 forecast models were explored.
They noted that it was crucial to consider the time lag between some environmental drivers of higher bacteria levels. For example, precipitation could take six days to lead to contaminated run-off reaching the beach. Other drivers had more immediate effects.
To evaluate the models’ performance, their predictions for historical timeframes were compared against previous bacteria measurements. Findings showed that certain model types had the greatest predictive ability, and overall performed as well as real-time models and the existing method used in California to predict beach water quality over short timeframes.
The major advantage of the forecast models is that they can effectively predict exceedance of bacteria thresholds three days in advance, by leveraging frequently monitored environmental data that are often available via the internet, such as tide level or precipitation. The researchers say that this study proves that these parameters, which influence bacteria fate and transport in the environment, can be used to make three-day forecasts; integrating this tool into beach management could enable better risk management. Forecasts could also inform proactive sampling if standard exceedances are predicted. Additionally, the framework could be extended to other phenomena such as algal blooms.
Footnotes:
- A review of the Bathing Water Directive (EUR-Lex - 32006L0007 - EN) is on-going. Find out more about the ongoing review and the consultation process on Have your say portal.
Source:
Searcy, R.T. and Boehm, A.B. (2022) Know Before You Go: Data-Driven Beach Water Quality Forecasting. Environmental Science & Technology. Available from: https://doi.org/10.1021/acs.est.2c05972
To cite this article/service:
“Science for Environment Policy”: European Commission DG Environment News Alert Service, edited by the Science Communication Unit, The University of the West of England, Bristol.
Notes on content:
The contents and views included in Science for Environment Policy are based on independent, peer reviewed research and do not necessarily reflect the position of the European Commission. Please note that this article is a summary of only one study. Other studies may come to other conclusions.
Details
- Publication date
- 12 April 2023
- Author
- Directorate-General for Environment